Scope
The scope of the IEEE Transactions on Cybernetics includes computational approaches to the field of cybernetics. Specifically, the transactions welcomes papers on communication and control across machines or between machine, human, and organizations. The scope includes such areas as computational intelligence, computer vision, neural networks, genetic algorithms, machine learning, fuzzy systems, cognitive systems, decision making, and robotics, to the extent that they contribute to the theme of cybernetics or demonstrate an application of cybernetics principles.
IEEE Transactions on Cybernetics replaced the IEEE Transactions on Systems, Man, and Cybernetics Part B: Cybernetics on January 1, 2013.
Editor-in-Chief
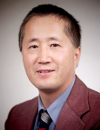
Articles
See more at IEEE Xplore